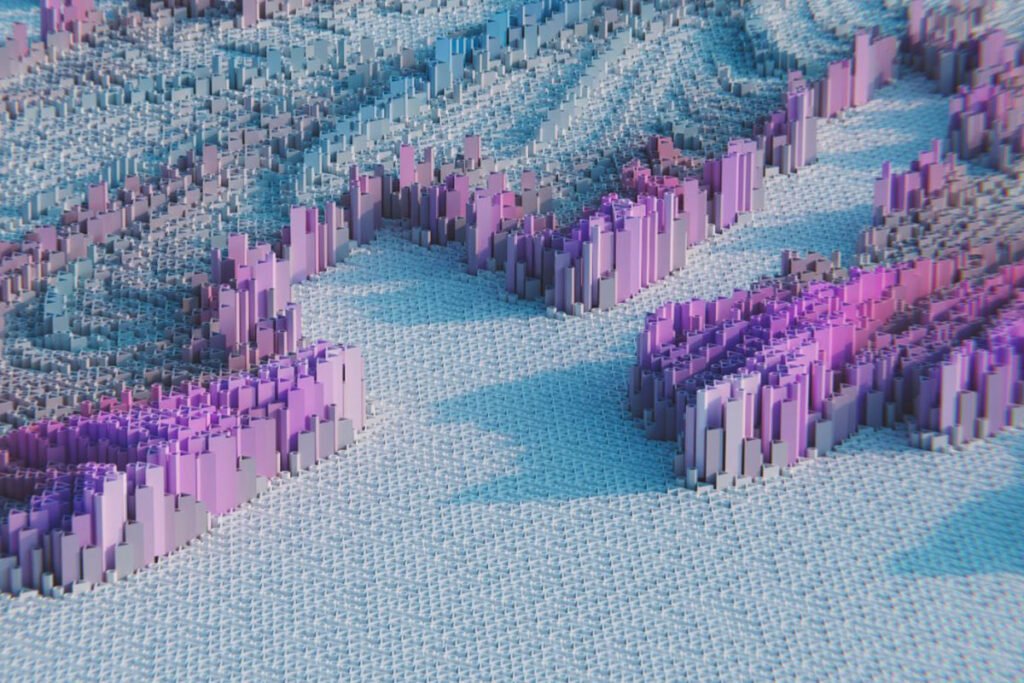
Â
I still remember the first time I encountered an AI model. I was working on a project that involved data analysis, and a colleague suggested using a machine learning algorithm to speed up the process. I was skeptical at first, but after seeing the results, I was hooked. The accuracy and speed at which the AI model processed the data was astonishing. But, as I delved deeper, I realized that there was more to using AI models than just pressing a few buttons.
Over the next few weeks, I spent countless hours learning about different types of AI models, their applications, and limitations. I read books, articles, and online tutorials, and even attended webinars and workshops. And yet, despite all the knowledge I gained, I still struggled to implement AI models in my own projects. It wasn’t until I started working on a project with a team of experienced data scientists that I finally began to understand the nuances of using AI models effectively.
One of the biggest challenges I faced was understanding the data requirements for different AI models. I had assumed that all AI models worked with any type of data, but I soon learned that each model has its own specific requirements. For example, some models require large amounts of labeled data, while others work better with unstructured data. And then there were the issues of data quality and preprocessing, which I had previously overlooked.
As I worked with my team, I realized that using AI models is not just about feeding data into a machine and waiting for results. It requires a deep understanding of the data, the problem you’re trying to solve, and the strengths and limitations of the AI model itself. It’s a iterative process that requires constant refinement and adjustment. And even then, there are no guarantees of success.
Despite the challenges, I was determined to master the use of AI models. I spent hours practicing, experimenting with different models and data sets, and seeking feedback from my colleagues. And slowly but surely, I started to see progress. My models became more accurate, my results more consistent, and my understanding of the data more nuanced.
But as I delved deeper into the world of AI models, I realized that there was more to it than just technical proficiency. I had to consider the ethical implications of using AI models, the potential biases and discrimination that could be perpetuated, and the impact on society as a whole. It was no longer just about solving a technical problem, but about using technology to make a positive impact on the world.
I remember one project in particular where we were working with a client in the healthcare industry. We were tasked with developing an AI model that could predict patient outcomes based on various factors such as age, gender, and medical history. But as we dug deeper, we realized that the data was biased towards a specific demographic, and that the model was perpetuating existing healthcare disparities. We had to go back to the drawing board and rework the model to ensure that it was fair and unbiased.
Â
Experiences like these taught me that using AI models is not just about technical expertise, but about responsibility, empathy, and a willingness to learn and adapt. It’s about understanding the impact of our work on others, and using technology to make a positive difference in the world.
As I look back on my journey, I realize that learning to use AI models is a continuous process. There is always more to learn, more to discover, and more to improve. But it’s a journey worth taking, because the potential rewards are immense. With AI models, we can solve some of the world’s most pressing problems, from climate change to healthcare to education. We can make a difference, one model at a time.